Recently, instructed by Prof. Wang Yongxiong and Prof. Tian Engang from the “High-end Equipment System Integration and Simulation Innovation” team in the School of Optical-electrical and Computer Engineering, two junior graduates Hu Chuanfei and Tang Zhenhui published their high-level paper in top journal: IEEE Transactions on Industrial Electronics (IF=7.503) and IEEE Transactions on Industrial Informatics (IF=7.377), and applied for their invention patents.
The team did their research on testing of vehicles and aero-planes. Faced with complexity of different kinds of alloy casting structures, and many inner defects of components and parts, X-ray perspective phenomenon based on casting structure, adopted advanced strategy of weak supervised monitoring, attention mechanism, digital process technology, accurately realizing the testing of hundreds of components and parts, and reducing the occurrence of major accidents.
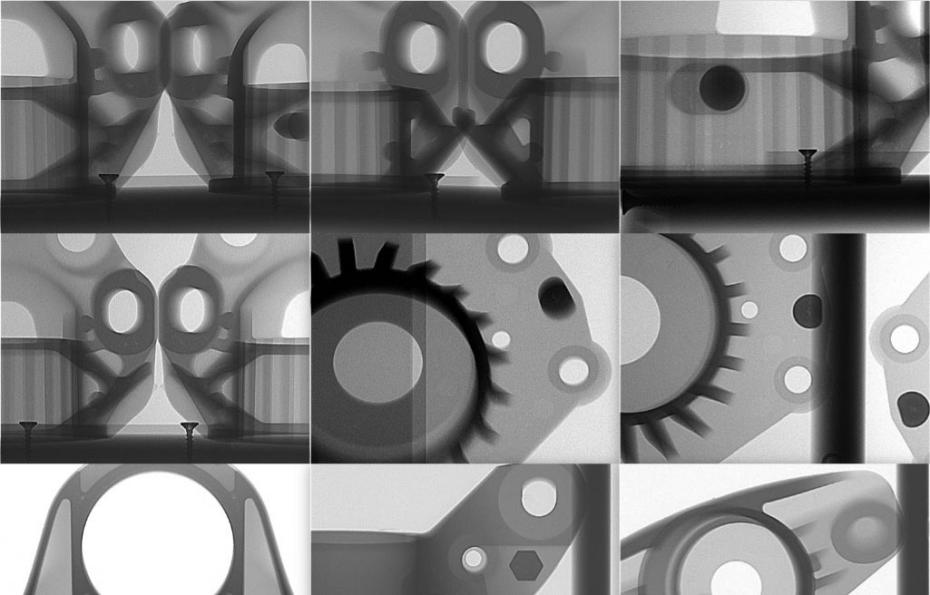
Hu Chuanfei published a research paper titled “An Efficient CNN Model Based on Object-level Attention Mechanism for Casting Defects Detection on Radiography Images”, and formulates AI solution program rather than human detection. An efficient CNN model, only trained with image-level labels, is first proposed for detection of tiny casting defects in a complicated industrial scene. Then, a novel training strategy, which can form a new object-level attention mechanism for the model during the training phase, was presented, and bilinear pooling is utilized to improve the model capability of detecting local contrast casting defects. Moreover, to enhance the interpretability, class activation maps (CAM) was extended to bilinear CAM (Bi-CAM) which is adapted to bilinear architectures as a visualization technique to describe the reason about the model output.
Link to the Paper: https://ieeexplore.ieee.org/document/8948332
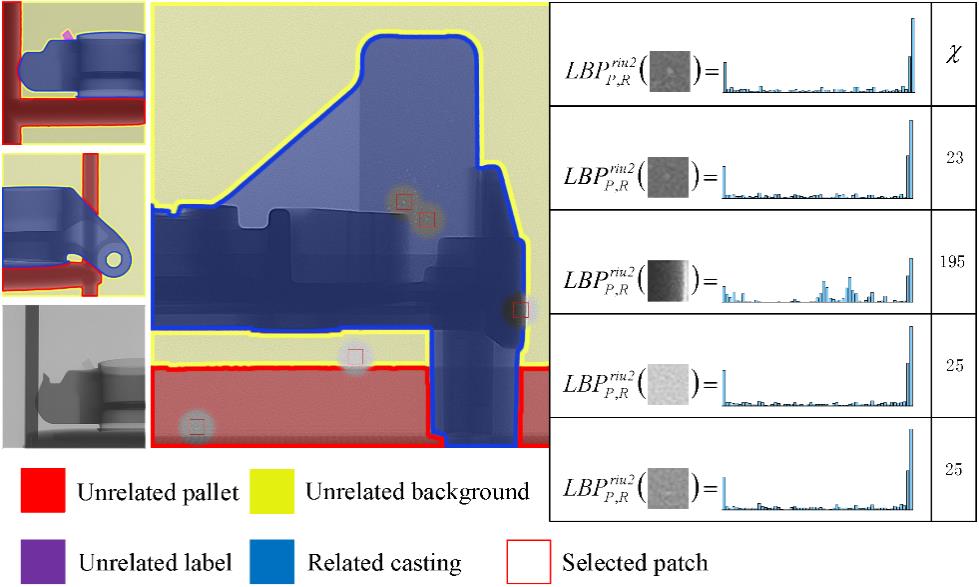
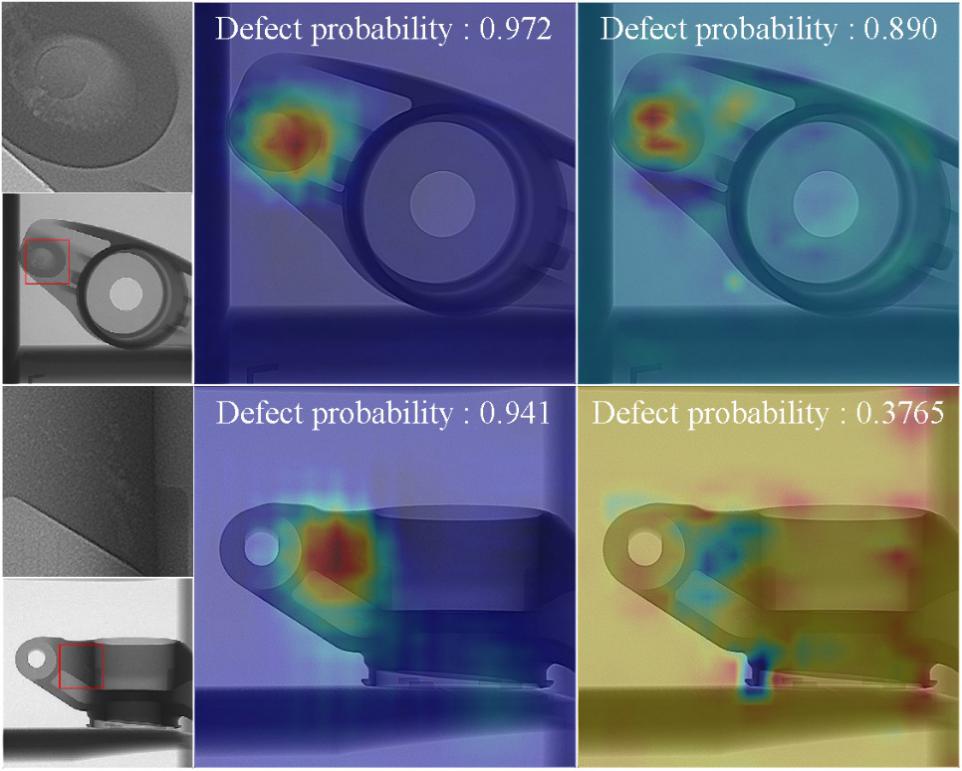
Tang Zhenhui published a research paper titled “Non-Destructive Defect Detection in Castings by Using Spatial Attention Bilinear Convolutional Neural Network”. This paper investigates the X-ray-image-based defective detection. The main contributions in this paper are twofold: 1) a new fullimage method is proposed to classify defective castings and non-defective ones; and 2) By combining two technologies, spatial attention mechanism and bilinear pooling used in deep convolutional neural networks, a new spatial attention bilinear convolutional neural network (SA-BCNN) is proposed to enhance the representation power of CNN.
Link to the paper: https://ieeexplore.ieee.org/document/9057424
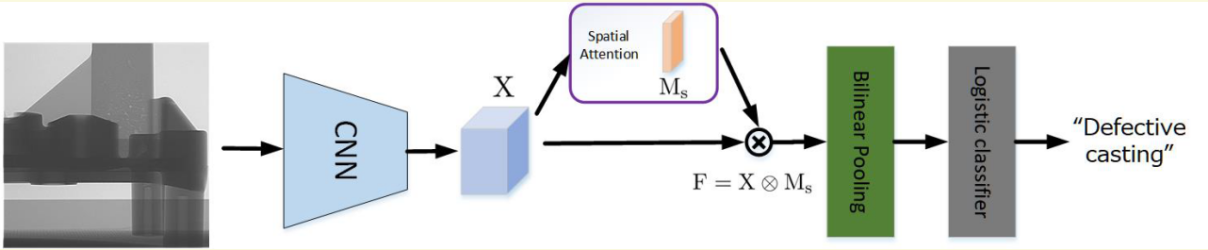
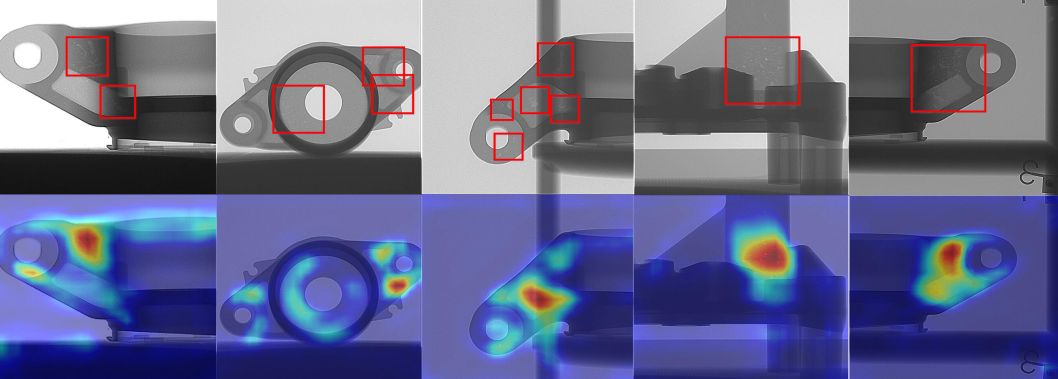