Recently, Hou Xuewen, a Ph.D student majoring in Biomedical Engineering from the School of Medical Instrument and Food Engineering, USST, published a paper titled (A One-Dimensional U-Net-Based Calibration-Transfer Method for Low-Field Nuclear Magnetic Resonance Signals)in international renowned journal Analytical Chemistry, (IF=6.986). Hou Xuewen and Professor Wang Xin were the first author, and professor Nie Shengdong was the corresponding author.
It is widely known that Magnetic Resonance Analytical Device can be affected by various factors such as magnetic field homogeneity, magnetic susceptibility and environment temperature, the spectrum signal tested in the same sample in one type of analytical device is of great difference, thus, the statistical analysis model established in a certain machine can not be removed to another. However, it is an expensive and time-consuming process to rebuild statistical analysis model in every analytical device.
As a result, in order to solve the fundamental scientific problem, based on Direct Standardization Algorithms theory,the team led by professor Nie Dongsheng proposed the iteration method to update and correct matrix, and this method groundbreakingly designed the one-dimensional U-Net calibration network that can be used in the calibration of low-field nuclear magnetic resonance signals.
This research used samples from two different systems, analyzed the correction differences between direct standardization algorithms and U-net network from three aspects like spectrum response, principal component analysis and statistical analysis model. The successful application of U-net calibration network in low-field nuclear analytical devices had laid a solid foundation for the deep learning of spectrum correction. Moreover, the method can also be popularized into the calibration of multidimensional imaging signal of Medical high-field magnetic resonance imaging devices produced by different manufactures, and thus effectively use AI to process polycentric medical imaging data.
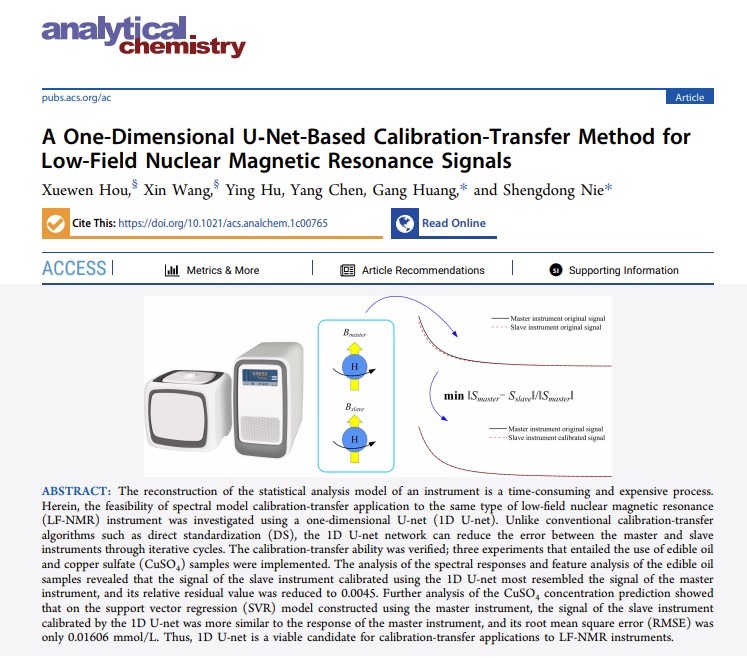
Link to the paper: https://doi.org/10.1021/acs.analchem.1c00765